As former teachers and school leaders, we understand the importance of reliability and accuracy in grading. That’s why we’re committed to the hard work of research, testing, and continuous validation to ensure our tools meet the...
EnlightenAI’s Commitment to Quality: Setting a New Standard in Grading Accuracy
- October 1, 2024
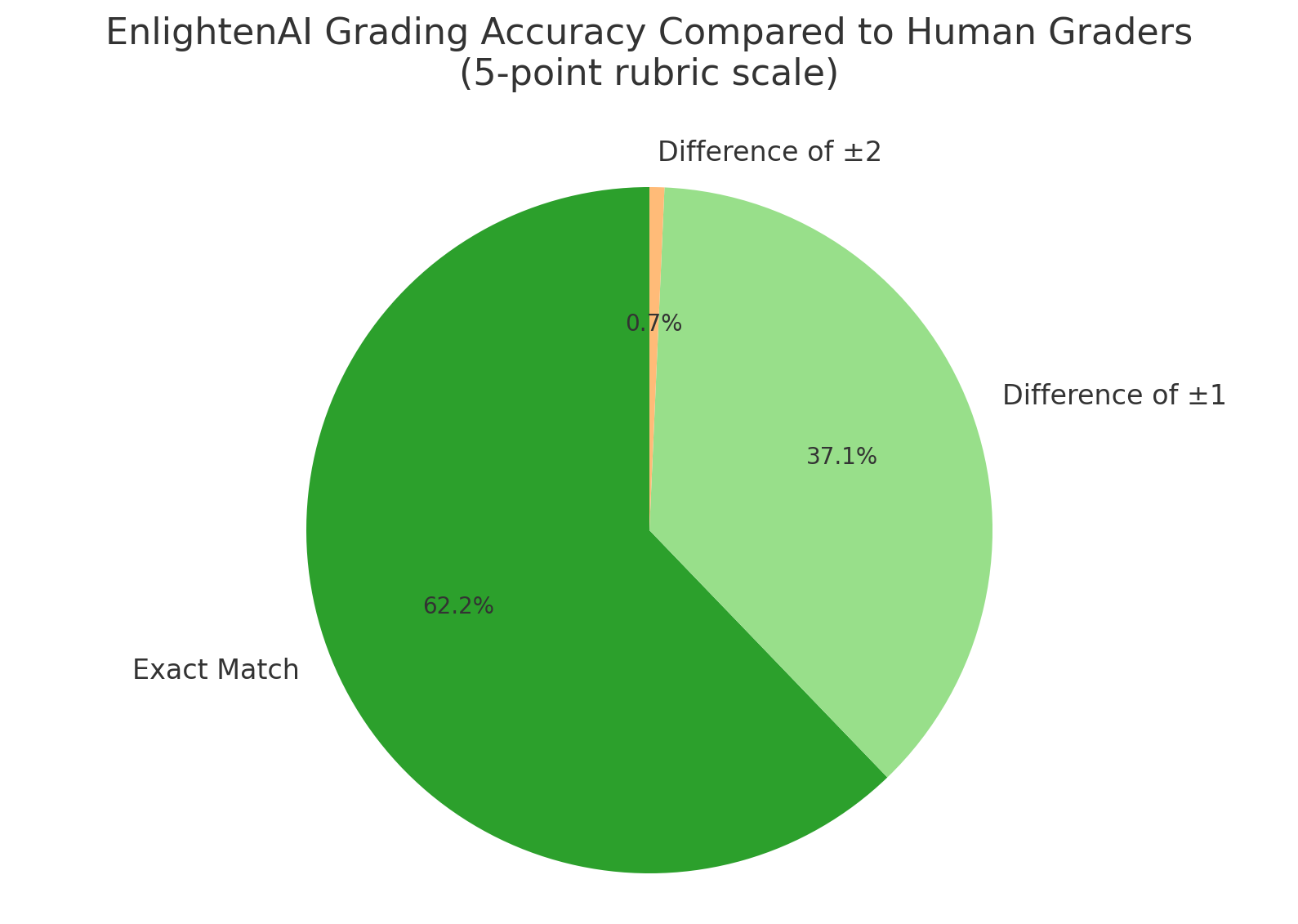
As former teachers and school leaders, we understand the importance of reliability and accuracy in grading. That’s why we’re committed to the hard work of research, testing, and continuous validation to ensure our tools meet the highest standards.
When we published our first case study with DREAM Charter Schools in New York, we set a benchmark for AI grading accuracy that beat ChatGPT and matched the best human scorers. But we knew we could push the boundaries even further. Last week, we implemented several improvements and ran the accuracy tests again. Truth be told, even we were surprised by the results.
A New High-Water Mark for AI Grading Accuracy
EnlightenAI has just set a new standard for grading alignment. In our latest round of testing, EnlightenAI matched the exact score given by DREAM’s human graders 62% of the time, and assigned a score within one point 99.3% of the time. These assessments were based on New York State’s holistic 5-point rubric for middle school ELA exams, which ranges from 0 to 4 points (inclusive).
How does this compare to human graders? It’s better than any human benchmarks we’ve found. EnlightenAI now meets or exceeds human thresholds for scoring accuracy, offering a level of precision that even well-calibrated human graders find difficult to match.
The Science Behind Our Success: Quadratic Weighted Kappa (QWK)
At the heart of our accuracy is the Quadratic Weighted Kappa (QWK) score, which reached an impressive 0.72 in our latest tests, indicating ‘substantial’ accuracy. Scores closer to 1 indicate higher levels of accuracy, and those closer to 0 signal the opposite. But what does this mean, exactly?
Here’s a quick way to understand how QWK works, and why it is so powerful as a measure of accuracy for grading. Imagine you’re playing a game of ‘Guess the Score’ with a friend (caution: the ‘game’ we’re about to describe is probably not your idea of fun). Your friend reads an essay and writes down a score, but you don’t know what score they gave it. Your job is to guess the exact score they gave based on the essay’s quality. Every time you guess the same score, it’s like hitting the jackpot—you’ve perfectly matched their judgment, and you’re awarded points for accuracy.
But here’s the catch: it’s not just about how often you get the score exactly right, it’s also about how close you are when you miss. If you guess a 3 and your friend gives the paper a 4, that’s a small miss, and your score will be penalized slightly. But if you guess a 1 and they gave it a 4, that’s a big miss—and big misses count much more against you. This is how Quadratic Weighted Kappa works, generally. It not only tracks how often EnlightenAI matches the exact score a human gave, but it also penalizes larger mistakes more harshly than smaller ones, making sure the final score reflects genuine skill and accuracy. It also adjusts for chance. Whether you’re working with a 5-point rubric or a 10-point rubric, the system takes into account that it’s easier to guess correctly when the scale is smaller.
That’s why a Quadratic Weighted Kappa score can’t be gamed. It accounts for chance, it penalizes large errors, and it makes sure you can’t cheat by guessing in the middle of the scale. This is what makes it the gold standard for measuring grading accuracy.
Why Our Quadratic Weighted Kappa Score of 0.72 is a Major Achievement
In our latest round of testing, EnlightenAI’s Quadratic Weighted Kappa score was 0.72, indicating ‘substantial’ accuracy. To put that in perspective, state-of-the-art Automated Essay Scoring (AES) systems, used for high-stakes exams like the SAT, typically score between 0.57 and 0.8. These systems are meticulously trained on lots of writing samples, but they’re rigid—they need new training for each new writing exam, and they don’t provide feedback.
EnlightenAI, on the other hand, is built for the classroom. It adapts to a wide variety of tasks and assignments while maintaining accuracy, without needing constant retraining. Our QWK score of 0.72 signals that EnlightenAI not only keeps up with the best AES systems but also offers teachers more flexibility and utility.
Figure 1. EnlightenAI Scoring Compared to Human Scorer (DREAM Charters)
The table above shows the results of comparing EnlightenAI scores with those actually given by DREAM graders for a given work sample. For example, if EnlightenAI assigned a score of 3 on the holistic rubric and DREAM actually gave the submission a 3, this counts as a perfect match. If, instead, EnlightenAI assigned a score of 4 while DREAM assigned a score of 3, this would count as a difference of +1.
Real Data, Real Challenges: Why This Result is Significant
Achieving this level of accuracy on real essays, graded by real educators, is no small feat. Classroom essays are messy—they come from diverse students with varied writing styles, and the scoring can be more nuanced and subjective than research settings. In a non-laboratory dataset like this, hitting human grading benchmarks is much harder to do than it might seem.
Moreover, none of the data we analyzed was anywhere on the internet. Large AI models like ChatGPT are often trained on massive datasets, some of which include public essay samples available online. Using data that is not in the public domain ensures our benchmarking test is a genuine measure of our AI’s accuracy, with no chance of the system “recognizing” the content from prior training data.
Figure 2. Performance Metrics of EnlightenAI Grading System Based on DREAM Dataset
Accuracy Statistic |
Percentage/Value |
Score within 1 point |
99.3% |
Score miss by >1 point |
0.7% |
Cohen’s Kappa |
0.42 |
Weighted Quadratic Kappa |
0.72 |
AI Grading Isn’t Magic—But It’s a Game-Changer
Let’s be clear: AI grading is not magic. We’re proud of what we’ve achieved, but there’s more work to do. Our research orientation requires us to point out that our testing was conducted on text-based responses with clear scoring guides, all built for middle school ELA tasks. We need to test our AI further in other content areas, curricula, and rubrics, and continue to push the boundaries of AI alignment.
Nevertheless, the idea that every teacher could have their own AI teacher’s assistant, one that learns from them, calibrates to their expectations, and drafts feedback in their voice, is game-changing. It shifts the teacher’s role from the repetitive physical work of grading to the more intellectual work of training their AI assistant, analyzing feedback for quality, and using data to drive instruction. This means teachers can focus more on helping students improve, which is ultimately what matters most.
Read more from EnlightenAI
Click below to check out other examples of EnlightenAI's impact.
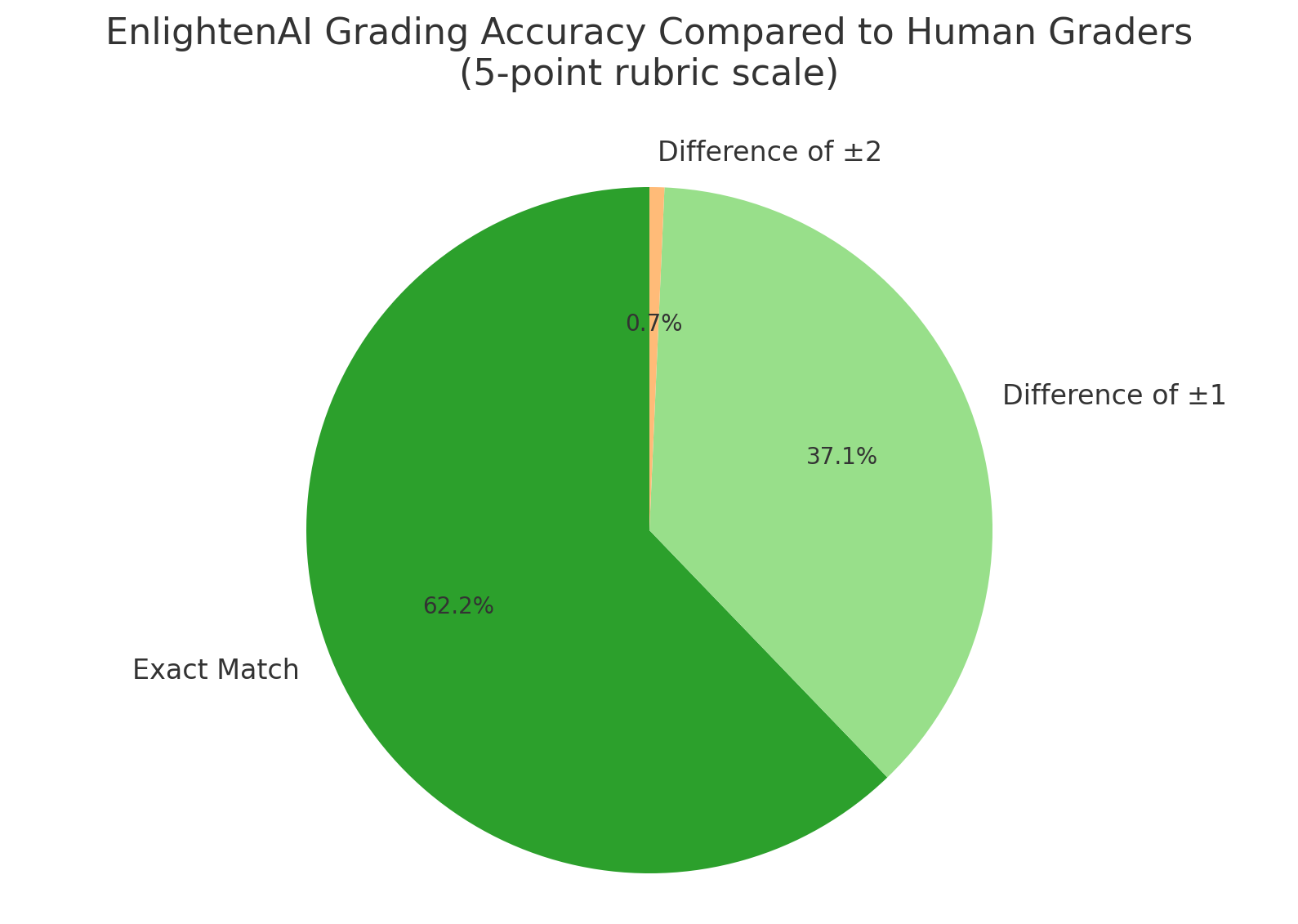
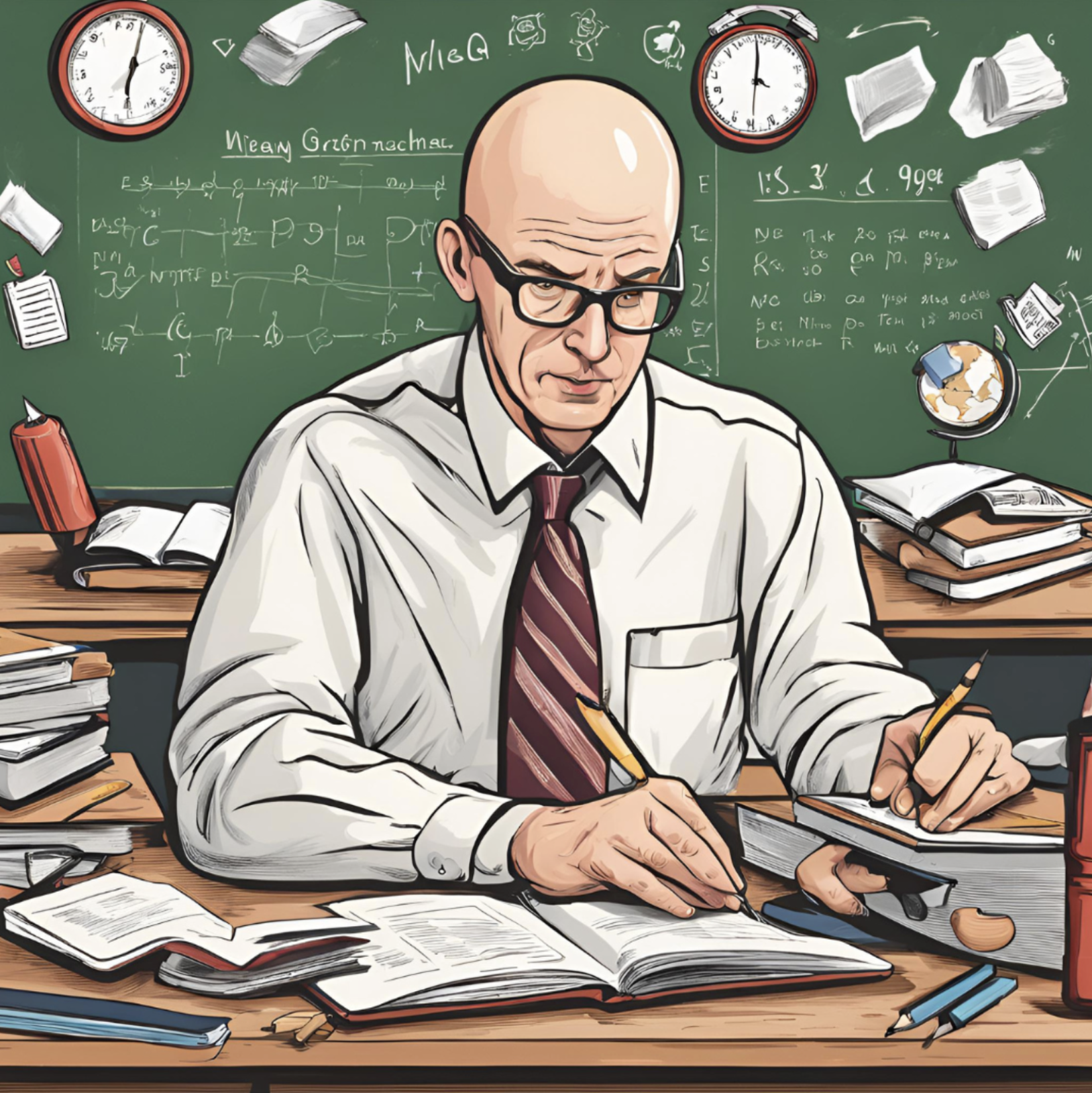
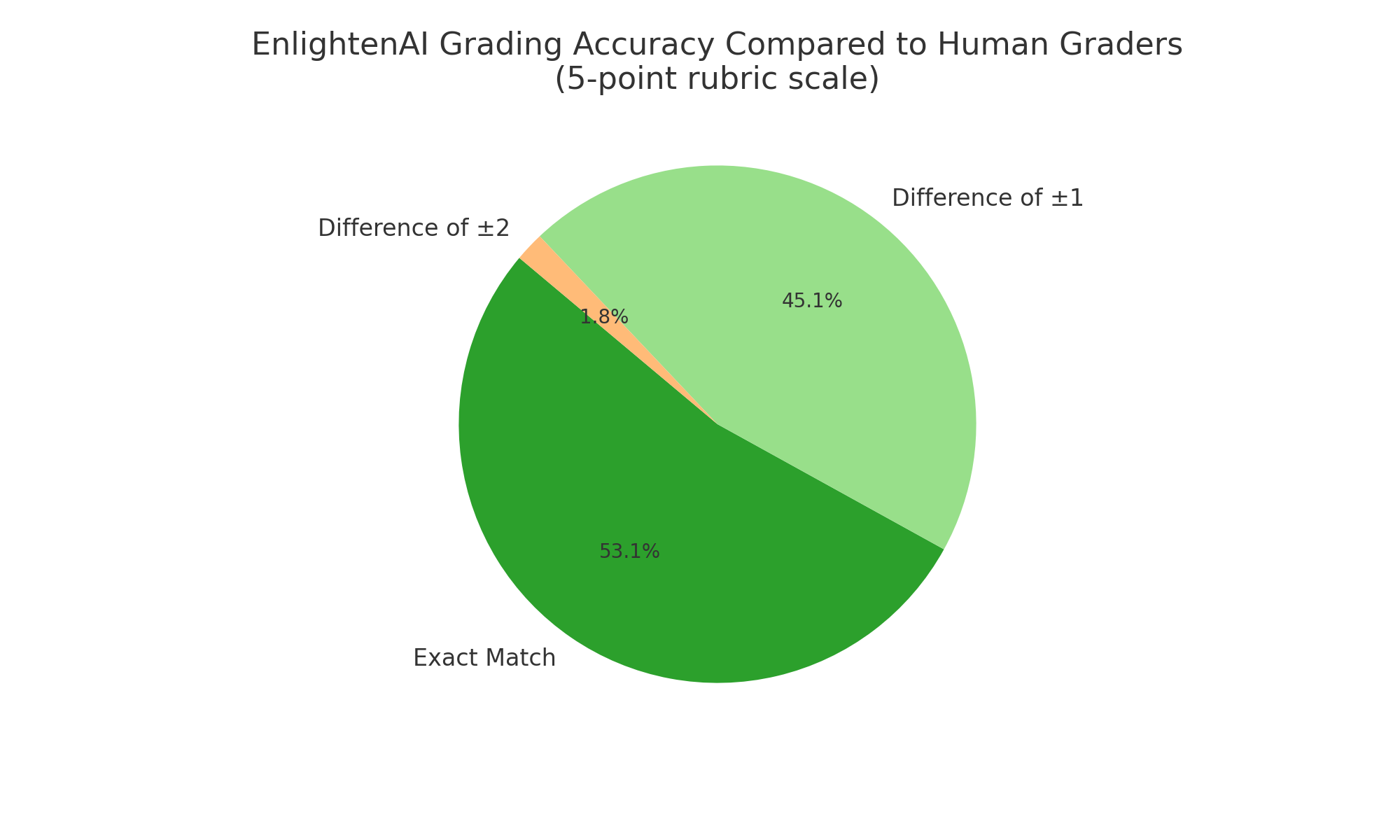